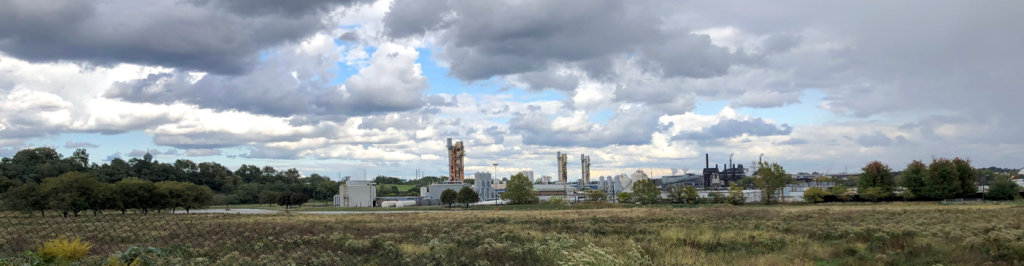
Since the FAIR data principles were proposed in 2016 by a diverse group of stakeholders in academia and industry, their adoption continues to grow. FAIR stands for Findability, Accessibility, Interoperability, and Reusability principles, meant to ease the ability of machines and humans to make informed use of data and maximize the value added of data analytics in a transparent, ethical way (Rocca-Serra et al., 2022).
When using social data, we propose an additional set of principles: FAIR2: Frame, Articulate, Identify and Report (also synthesized in this report). This framework recognizes that data do not speak for themselves, that observational data reflect discrimination in society, and that an explicit framework to identify and address discrimination biases will further data science’s potential to advance social justice.
“The invisible layers of racial ideology packed into the statistics, sociological theories, and the everyday stories we continue to tell about crime in modern urban America are a legacy of the past. The choice about which narratives we attach to the data in the future, however, is ours to make.”
Khalil Gibran Muhammad
Frame
Frame metadata and data with historical context and experiential knowledge of those represented in the data. The way in which administrative data capture individuals’ interactions with systems spanning from healthcare to policing is permeated by discrimination.
Articulate
Articulate the general model as a causal graph to explicitly state model assumptions (background knowledge) and hypotheses about the role of discrimination in the social phenomenon studied. It has been said that the logic of inference in policy analysis can be summarized as “assumptions + data = conclusions” (Manski, 2013). In other words, the data do not speak for themselves; assumptions can be a source of subjectivity in inference. Directed Acyclic Graphs (DAGs) are a collection of nodes and directed edges connected under certain conditions to represent a causal model (Pearl, 1995). DAGs make explicit the assumptions embedded in the model, helping to clarify the source of these assumptions (what knowledge and whose knowledge?) and their implications for model estimates (Pearl & Mackenzie, 2018).
Identify
Identify bias embedded in the data and variables of interest, aiming to minimize bias and report on limitations due to bias. Here we draw from the work of (Kleinberg, Ludwig, Mullainathan, & Sunstein, 2018) and (Lundberg, Johnson, & Stewart, 2021) to systematically analyze potential biases in the estimand and choice of variables used in the model. We set out to answer the following questions: (1) What is the unit-specific quantity of interest -USQ- and the target population? (2) What biases may be introduced by each variable and by using measured versus desired variables? (3) What is the role of variables representing the sensitive attributes (subject to discrimination) in the model? (4) For whom is the USQ missing in the data (selective labels problem; collider bias), why, and how will this affect model estimates?
Report
Share findings and seek feedback from members or agencies in the community who have experiential knowledge of the social issue analyzed. Engaging community stakeholders in analyzing and reporting findings completes the circuit of utilizing data without losing its human context.
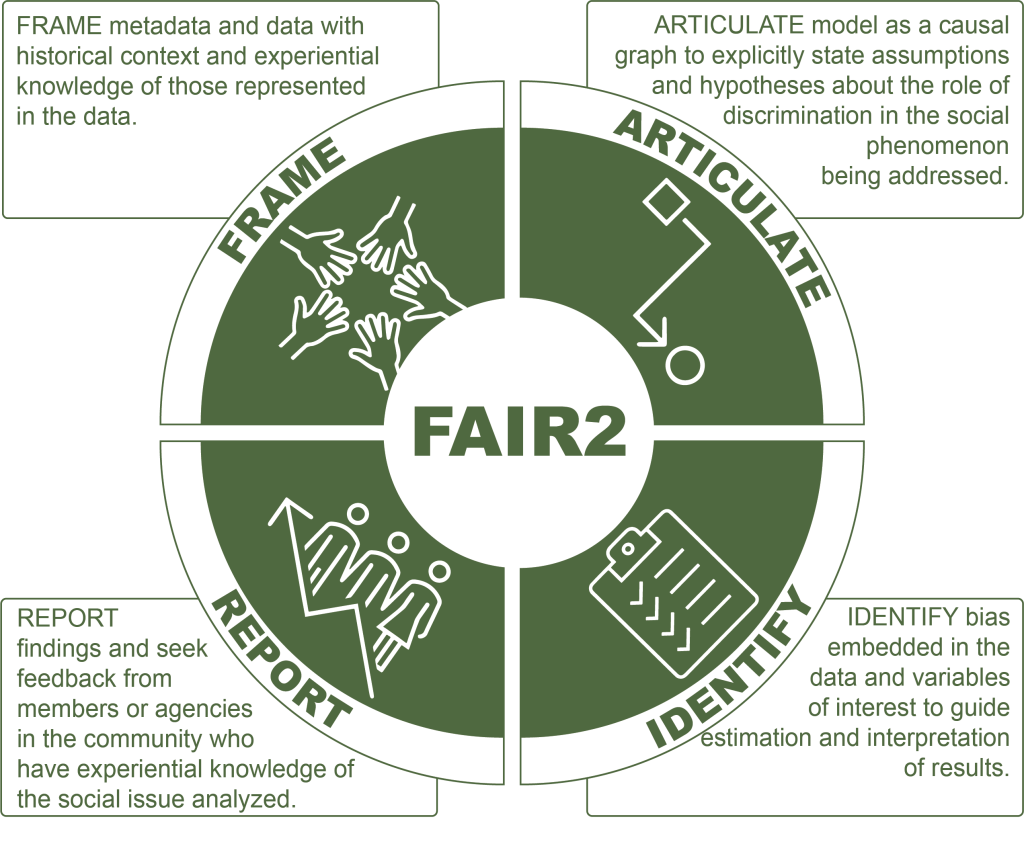
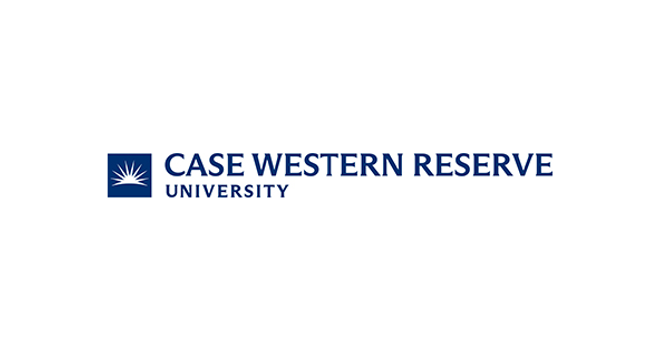
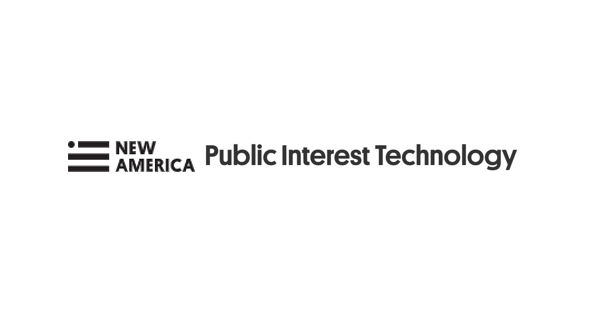