We illustrate the use of the FAIR2 framework with an analysis of recertification in the Supplemental Nutrition Assistance Program (SNAP), one of the main public assistance programs in the United States. SNAP is offered as a food voucher via Electronic Transfer Cards, conditional on meeting low-income thresholds that vary by state. SNAP is among the strongest programs in the US social safety net, with a countercyclical multiplier effect during economic downturns (Canning & Stacy, 2019). States require individuals to follow a recertification process every 6 to 12 months involving detailed proof of income and an interview. Research suggests that up to half of beneficiaries who exit SNAP within their first year were still eligible (Gray, 2019). Failing to recertify despite qualifying –here denoted as FR– is costly to individuals and administrative agencies.
Researchers have sought to study who is affected by FR and what can be done to reduce the rate of FR.
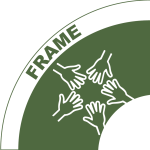
Following FAIR2, we frame or contextualize the administrative data with experiential knowledge from subject matter experts: Data Chat participants who have experienced the SNAP recertification process.
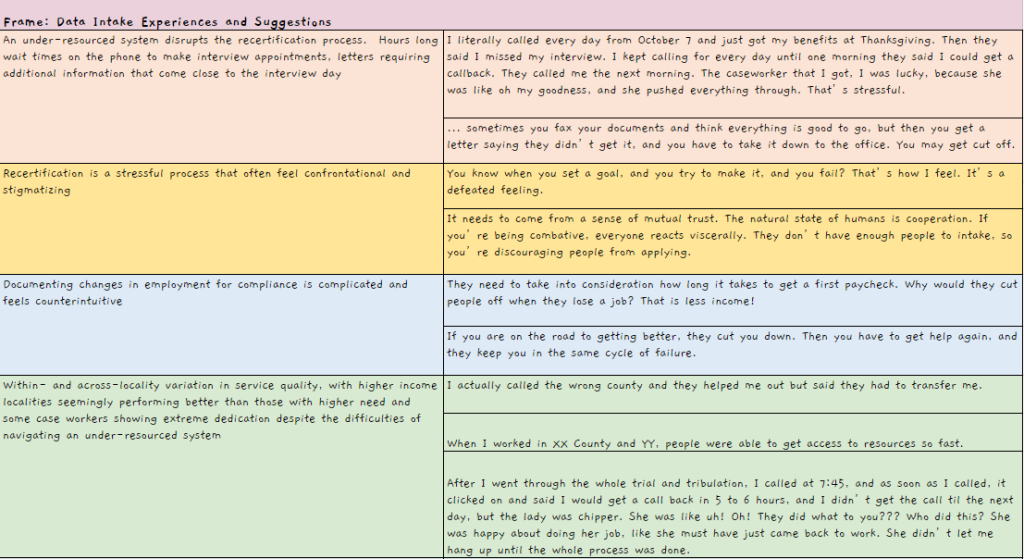
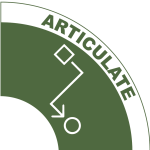
Insights from experiential subject matter experts (Data Chat participants) inform the modeling of what prevents people from successfully recertifying to receive SNAP benefits. The table illustrates how participants’ ideas informed the causal pathways seen in the Directed Acyclic Graph (DAG) below.
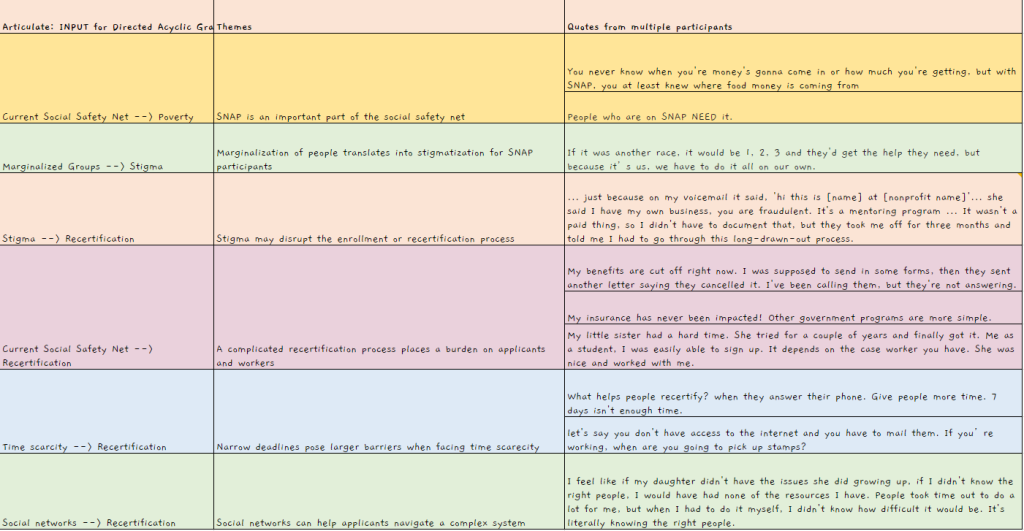
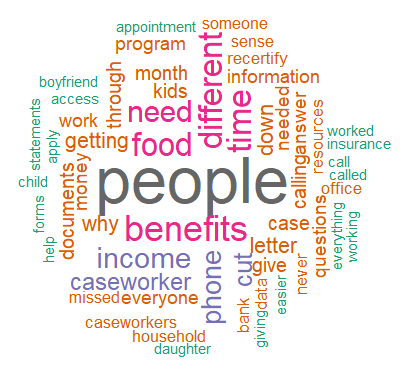
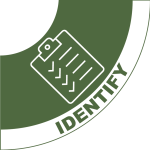
We answer the following questions to explicitly identify bias embedded in the data and variables of interest, and report on limitations due to bias.
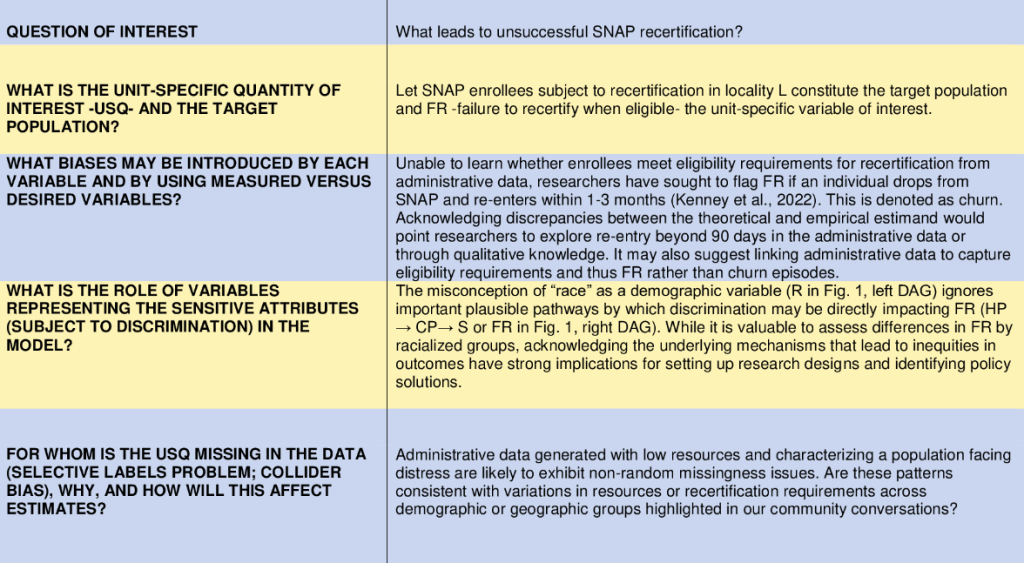
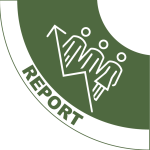
Reporting for this process involved a large group meeting. In the case of group meetings, it is important to make sure everyone has a chance to voice their thoughts.
Working models, important themes, and lessons learned about the structure of the data chats themselves are shared and feedback is solicited from our collaborators.
This feedback is dynamic and can change the model and insights highlighted. It is of the utmost importance that community collaborators feel that their voices are captured accurately. This was also an opportunity for collaborators to provide valuable feedback for action beyond the Data Chats, which we summarize here.

Click to Enlarge
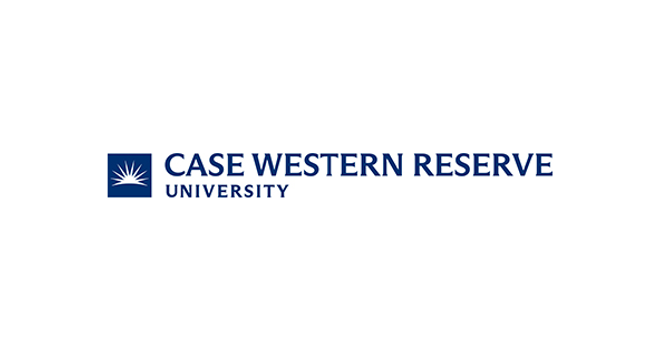
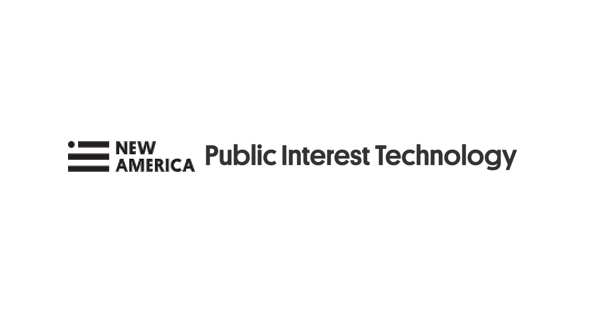